Are owners and operators accurately accounting for energy losses in a wind farm?
A case study demonstrating how a machine learning framework can be used to accurately account for energy losses in a wind farm.
Problem
A key issue for wind turbine owners and operators is understanding the causes of energy loss and the resulting revenue loss. Wind farms can range from very small with just a few turbines to very large with more than 100 turbines. They can also vary from being located in flat terrain to a very complex terrain. In last few years, with re-powering of older sites, wind farms with mixed turbine types are also on the rise. It is critical for owners and operators to understand the true energy loss and the reasons for the losses. This applies equally to wind farms that are self-perform or under full-wrap supply agreement.
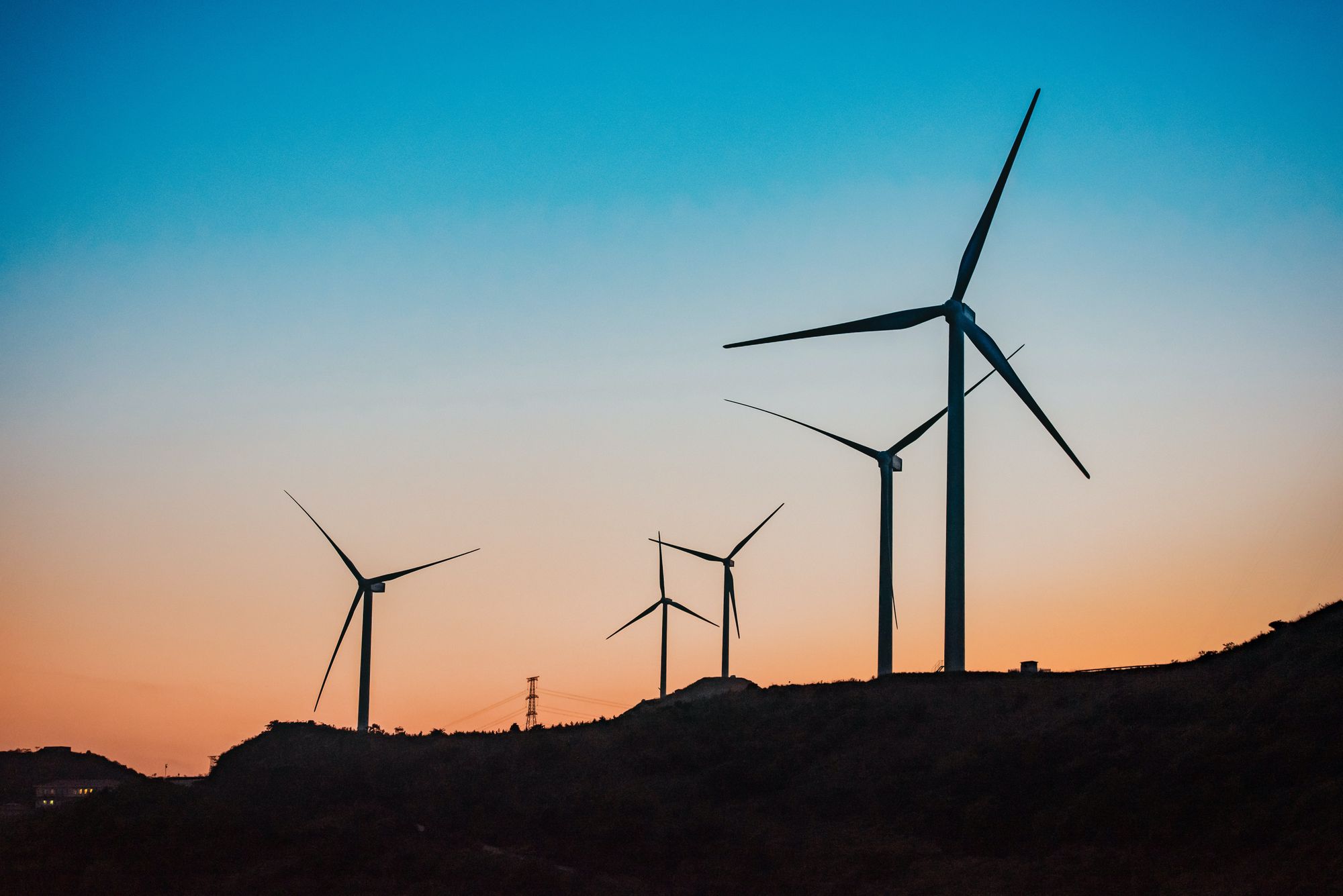
Past Approaches
The most common method for calculating lost energy is using the nearest neighbor to calculate the energy loss during downtime. This method provides accurate results under a very narrow set of cases such as a wind farm with flat terrain and significant distance between the turbines. For more typical wind farms with wake effects & complex terrain (and recently mixed turbine wind farms), the calculated energy losses using this method can be off by as much as 10%. This affects the customer bottom line as it affects the calculation of Availability Damages or Availability Bonuses.
Ensemble Solution
Accurate calculations of project energy losses are critical for wind farm owners with or without an OEM warranty. Fortunately, wind farms are data rich, and physics informed machine learning approaches provide a framework to calculate these losses much more accurately. One Ensemble customer owns and operates more than 1.0 GW of wind assets with wind farms ranging from just a few MW to more than 200 MW. Their fleet also consists of multiple OEMs. Their challenge was to provide a unified view for all of their assets and reconcile energy losses provided by different OEMs. In addition, the OEMs were only providing energy loss for downtime related to turbine unavailability, which leads to a complete lack of visibility into lost revenue because of BOP or contractually excluded downtime. The plot below shows how traditional energy loss calculations can have significant errors and how Ensemble’s machine learning based method improve the accuracy of loss calculations.
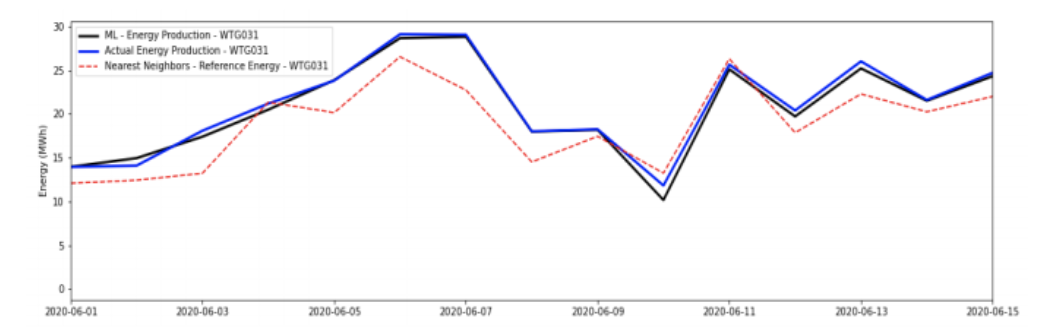
Another use case of Ensemble's ML based energy calculation method is to calculate energy loss due to BOP issues such as a feeder failure or substation outage. In one instance, a site had a section of the wind farm down for more than a month because of an electrical outage. The OEM did not report the energy loss as it was outside their scope. In addition, there was no SCADA data available for this timeframe for the affected turbines. However, Ensemble's ML based method was able to calculate the energy loss for the affected section of the project. The energy loss was significantly higher than what owner's method calculated and reduced the energy based BOP availability by over 8%. Besides an accurate accounting of the energy loss, this guided the owner's decisions for spare parts inventory planning.
Accurately calculating losses due to grid curtailment is also an important issue for operators. Wind farms may be curtailed for various reasons with grid curtailment being the most common issue. One wind farm monitored by Ensemble is located in an ISO with significant grid curtailment, especially during certain months of the year. Our customer was using the OEM estimate of energy loss. When Ensemble reviewed the calculations, the estimate was based on an average wind speed across the site using a single power curve. This wind farm has over 75 turbines and more importantly a mix of turbines, so this calculation methodology was bound to be incorrect. Ensemble's data driven method allowed our customer to accurately calculate the energy loss from curtailment and get the accurate curtailment compensation from the off taker. The OEM calculation underestimated the losses by nearly 10% over one year.
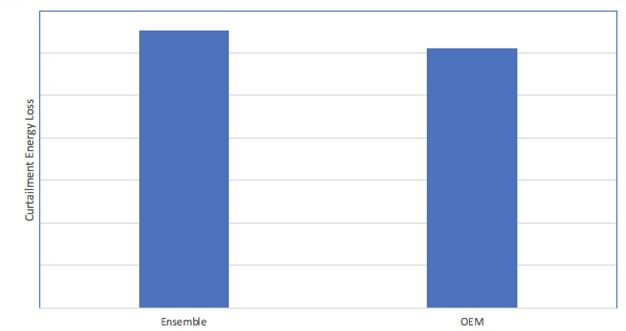
Benefits
More accurate energy loss calculations such as those provided by Ensemble’s machine learning based methods help operators receive the true value from their O&M contracts. They also help owners understand where energy is being lost, so they can focus their efforts on corrective actions with the highest ROI.